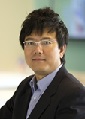
Da Qi Ren
Futurewei Technologies, USA
Title: Service-Oriented Models for Cloud Based Big Data Analytics
Biography
Biography: Da Qi Ren
Abstract
A growing number of enterprises use cloud computing to build their big data projects because the cloud offers a cost-effective way to support big data technologies and the advanced analytic applications that respond to real business needs and drive commercial values. Cloud computing provides a wide range of infrastructure and software services and manages large numbers of virtualized resources, which makes advantageous computing paradigms available for big data. A modern cloud can behave virtually like a local homogeneous computer cluster, providing high performance, data intensive computing platforms for public use. These platforms can potentially enhance business agility and productivity while enabling greater efficiencies and reducing costs. In this work, a service oriented hierarchical model is introduced to assist the assurance of the high performance business service over the virtual clusters on a cloud where the data intensive computing paradigms are deployed. This model is especially illustrated by exploiting and modeling the business workload characterization, constraints in software stack and low-level distributed resources. The modeling mechanisms, including the primitives and functionalities, are formulated. The service-oriented model aids in systematic and hierarchical development of global optimization for big data analytics on a cloud. It is suitable for government, education, finance, medical, telecom and other industries and for all types of offices, including branches, call centers and mobile office situations. A cloud solution for big data is an end-to-end solution covering hardware, software, network, terminal, security, consulting and design services. Cloud servers are composed of a cloud OS and virtualized platforms. Through centralized managing and sharing of computing and storage resources, the cloud platform helps customers solve the problems of traditional clusters and allows them to enhance information security, improve O&M efficiency and create a truly mobile office while improving service reliability. Cloud hardware integrates computing, storage and network. The computing devices usually include a multi-core CPU, a GPU, an FPGA and other multiprocessing facilities. Smart storage engines, intelligent networks, SSD caching mechanisms and other innovations work together to achieve high performance. Systems are designed as pre-validated infrastructure under unified physical and virtual resource management. Big data analytics on a cloud are expanding rapidly in terms of the increase in the workload and variety of businesses on a cloud. For example, some of the big data workloads have more branch operations, some are data-movement-dominated-computing, and some have larger instruction footprints. Typically, Hadoop- and Spark-based big data workloads have higher front end stalls, and complex big data software stacks fail to use state-of-practice processors efficiently. The architectural designs of the cloud services have to meet the performance requirements of the specific business characterizations. Service models developed by analyzing the factors of workload, cost, security and data interoperability are vital to performance. Depending on the usage scenario and the performance requirements, the best use of the cloud platform may be to focus on analytics as a service (AaaS). Cloud service models can help accelerate the potential for scalable big data analytics solutions. Cloud based big data analytics is not a one-size-fits-all solution. Organizations using cloud infrastructure to provide AaaS have multiple options. Businesses with varying needs and budgets determine the strategies to create a service model in cloud environments. Computing power and storage capacity via cloud services for certain analytics initiatives provide added capacity and scale as needed. Based on the service model for a business on a cloud, data localities need to be designed to analyze the data either in a cloud data center or in edge systems and client devices. By focusing on handling the critical configurable design constraints at each level of a cloud platform, optimized big data analytic services can be approached based on the above service-oriented model to achieve the best possible performance. The model allows obtaining design characteristic values in the early design stage, thus benefitting the cloud administrator by providing the necessary workload information for choosing the best computing, storage and network alternatives. The model is embedded in the management system: it allows access to everything, including switches, virtual machines, storage volumes, applications provisioning, automation and security. The model supports the suggestion of flexible compute/storage configurations, scalabilities, configuration/expansion on demand and improvement of storage I/O performances. Working together with the cloud management system, the service-oriented model virtualizes and schedules computing, storage and network resources and provides services such as elastic computing, load balancing and virtual private cloud. Finally, the hardware software synergy to achieve application optimization.